Insights and Highlights: Self-Regulatory Approaches to AI Governance
In the absence of AI regulation, can industry self-regulation help address consumer trust?
Insights and Highlights Self-Regulatory Approaches To AI Governance
Subscribe to Newsletter
Related Posts
Center for Ethics Inaugural AI Ethics Strategy Fellow
View DetailsAdaptable Artificial Intelligence
View DetailsUnlock the Power of Trust with The Trust Certificate Program
View DetailsEthics In Financial Services Insights
June 26, 2024
At the American College Center for Ethics in Financial Services’ AI Ethics in Financial Services Summit on April 2, 2024, Sophia Duffy, JD, CPA, AEP® moderated the "Self-Regulatory Approaches to AI Governance" panel. Panelists included Anthony Habayeb, co-founder and CEO of Monitaur, and Reva Schwartz, research scientist at the National Institute of Standards and Technology (NIST). The panel explored artificial intelligence (AI) governance, emphasizing self-regulation, risk management, and the intersection of technical and social considerations.
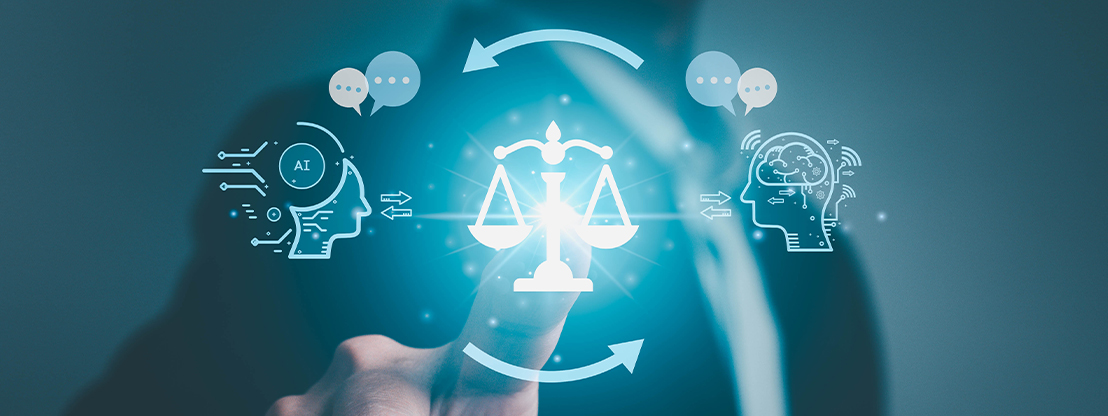
The panelists emphasized that good model development practices, irrespective of regulatory requirements, lead to better performance and predictability in tech investments. Companies implementing self-governance ahead of regulations often perform better by integrating risk management with economic considerations. The NIST framework, “Towards a Standard for Identifying and Managing Bias in Artificial Intelligence,” addresses both technical and social impacts of AI, ensuring comprehensive governance.
Mandated by Congress in 2021, NIST developed a risk-based framework for managing AI models and practices. This flexible resource aids organizations in governing, mapping, measuring, and managing bias in AI. By focusing on governance, policies, procedures, and organizational culture, organizations can take a comprehensive approach to this challenge. By taking a proactive approach to governance, the aim is to help organizations promote trustworthy AI practices, including model validity, reliability, security, resilience, explainability, accountability, transparency, privacy, fairness, and bias mitigation.
The panel also discussed the relationship between federal and state initiatives and the role of self-regulation in AI governance. One panelist mentioned the AI Executive Order's contribution to defining real risks and the ongoing work on an AI risk management profile for generative AI. Another stressed the need for clear documentation and repeatable practices to provide assurance to partners.
The conversation also covered the challenges of accountability within organizations, highlighting the need for a cultural shift towards responsible AI use. The panel emphasized the importance of integrating AI risk management with broader enterprise risk management frameworks and adopting a shared responsibility model with third-party vendors.
Looking forward, one panelist predicted that AI risk management would become a distinct job category, with an increased focus on the societal impacts of AI. Another anticipated a progressive impact on software quality control driven by AI, leading to more regulated software development practices.
In summary, the panel highlighted that given the evolving regulatory landscape there is a need for clear and transparent AI governance practices, as well as the importance of interdisciplinary collaboration and cultural shifts towards responsible AI use.
To learn more about AI in financial services, you can explore further with research from the Center for Ethics in Financial Services.
Related Posts
Center for Ethics Inaugural AI Ethics Strategy Fellow
View DetailsAdaptable Artificial Intelligence
View DetailsUnlock the Power of Trust with The Trust Certificate Program
View Details